Nvidia found itself in an opportune position with the surging demand for its GPU chips, driven by the resource needs of generative AI models. Nonetheless, what if there existed an alternative chip with comparable capabilities but at a more affordable cost? Lemurian Labs, a budding company comprising former staff from Google, Intel, and Nvidia, is embarking on the quest to develop precisely such a solution.
This is indeed a moonshot idea, and it takes a lot of time and money to bring a chip innovation to market, but when it comes from entrepreneurs with a certain reputation, investors are prepared to take a chance on this kind of idea. The business has just disclosed a $9 million seed financing.
The startup aims to create a fresh processor and software solution designed to simplify, expedite, reduce costs, and, in the long run, have a more environmentally sustainable approach to executing AI tasks.
Lemurian elucidates that the essence of computing can be distilled into three fundamental elements: “There’s mathematics, there’s memory, and then there’s the aspect of data movement,” almost akin to delivering an expert lecture on computer architecture. Interconnects are the objective. Data is therefore stored in memories, transferred across a network to a math unit, where it is changed, and then written back to memory. Data must travel, hence that is the conventional point in design, according to Dawani.
Lemurian intends to respond to this by altering the math on the chip, an enormous job. According to Dawani, engineers decided to utilize a floating point technique in the early stages of chip development because no one could make a logarithmic approach function. He asserts that the issue has been resolved by his business.
The added benefit of a log number system is that it converts all those costly operations into adds and subtractions, which are very cheap hardware operations. Thus, you conserve space and energy while gaining speed. Additionally, you gain a little in terms of exactness or precision, both of which are very appealing when attempting to reduce the processing cost for sizable language models.
“And the dynamic range becomes better and better for the same number of bits as you raise the number of bits, which is just interesting. Now, the number system is a major factor in enabling us to explore the design we did since without it, you would be subject to the same restrictions.
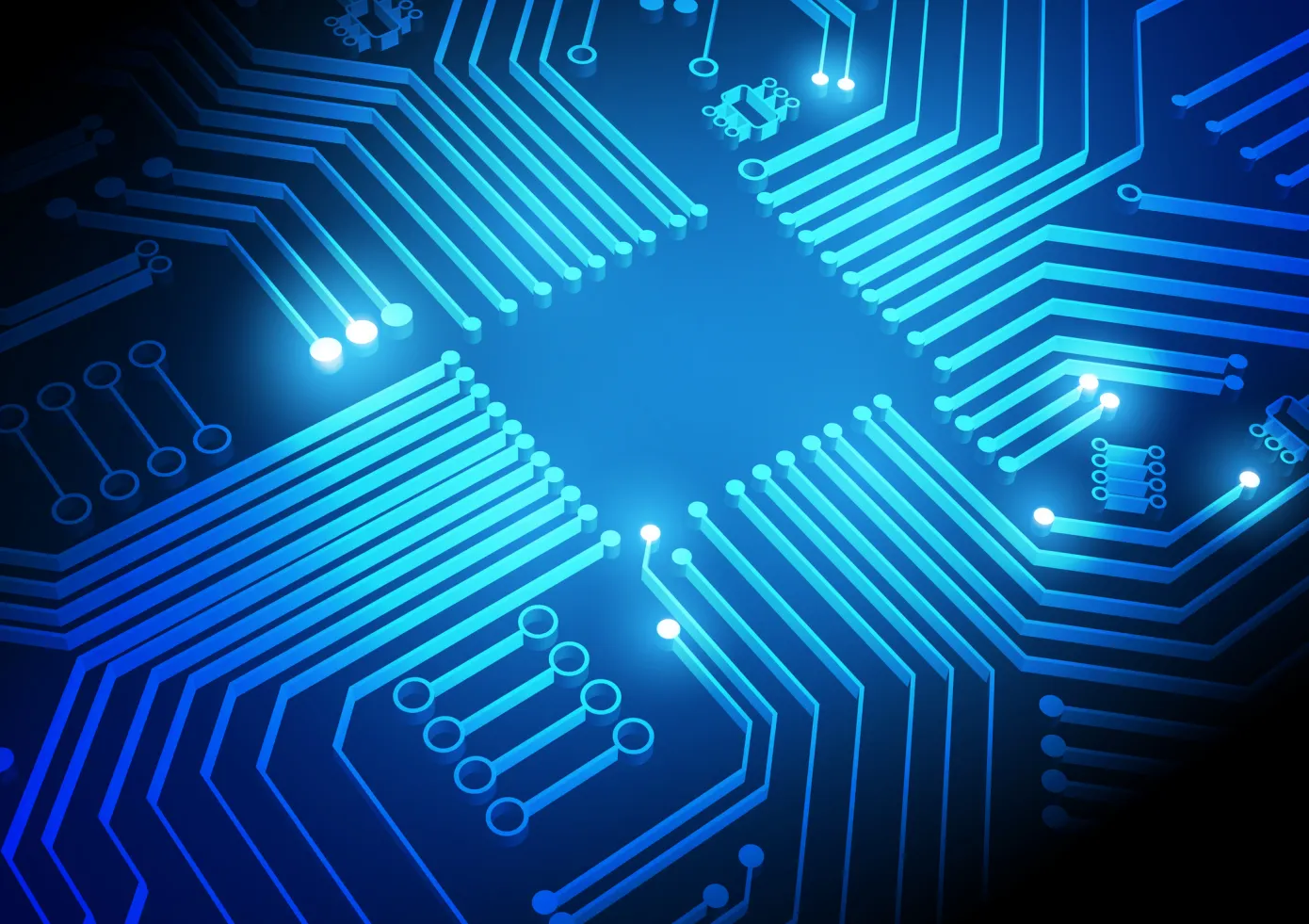
They are adopting a cautious approach and releasing the software first. They aim to have it generally available in Q3 of next year. The objective is for the hardware to catch up in the subsequent years, although this endeavor is expected to be significantly more challenging and will necessitate additional time and financial resources for development, manufacturing, and production testing.
At present, the company boasts a workforce of 24 individuals, the majority of whom are highly skilled technical engineers with prior experience on similar projects. While the current pool of potential candidates is limited, the company anticipates bringing on board an additional six hires in the upcoming months. If all proceeds as envisioned and they secure a Series A funding round, they have plans to expand their team by an additional 35 individuals in the following year.
Oval Park Capital spearheaded the $9 million investment, which also included contributions from Alumni Ventures, Raptor Group, Good Growth Capital, and others.
It will be extremely difficult and expensive to develop a company like this and bring the technology to market, but if they succeed in what they say, it may make creating generative AI models (and whatever follows next) far more affordable and effective.
Engineer | Content Writer
Want to be a catalyst for a positive change in the world