Introduction:
The demand for generative AI, which is frequently trained and operated on GPUs, is outpacing the supply of GPUs. According to reports, Nvidia’s top-performing CPUs won’t be available until 2024. Recently, the CEO of chipmaker Amazon Unveils New Chips for Training TSMC expressed less optimism, speculating that the shortfall of GPUs from Nvidia and its competitors would last until 2025.
Companies that can afford it, i.e., the big tech companies, are designing — and sometimes making it available to customers — bespoke CPUs intended to develop, refine, and commercialize AI models to reduce their need for GPUs. Among those companies is Amazon, which debuted the newest iteration of their chips for model training and inferencing (i.e., running taught models) today at its annual re: Invent conference.
AWS Trainium2, the first of two, was introduced by Amazon in December 2020 and is intended to provide up to 4x higher performance and 2x better energy efficiency than the first-generation Trainium. Tranium2 will be accessible in the AWS cloud in EC Trn2 instances in 16-chip clusters. Tranium2 can scale up to 100,000 chips in AWS’ EC2 UltraCluster offering.
According to Amazon, a single Trainium processor can process 65 exaflops of data, or 650 teraflops, out of 100,000. The terms “exaflop” and “teraflop” describe the number of compute operations a chip can do in a second.) That quick calculation on the back of the napkin could be more precise due to various complicating considerations; however, if a single Tranium2 chip can produce ~200 teraflops of performance, that is significantly more than Google’s specialized AI training chips from around 2017.
According to Amazon, a 300 billion parameter AI large language model may be trained in weeks rather than months using a cluster of 100,000 Trainium chips. A model’s components learned via training data are called “parameters,” they specify the model’s ability to solve a specific task, such as writing code or text. That is almost 1.75 times larger than GPT-3 from OpenAI, the model used to create GPT-4, a text generator.
AWS compute and networking vice president David Brown stated in a press statement that “silicon underpins every customer workload, making it a critical area of innovation for AWS.” “[Tranium2 will help customers train their ML models faster, at a lower cost, and with better energy efficiency, given the surge in interest in generative AI.”
Amazon Unveils New Chips for Training:
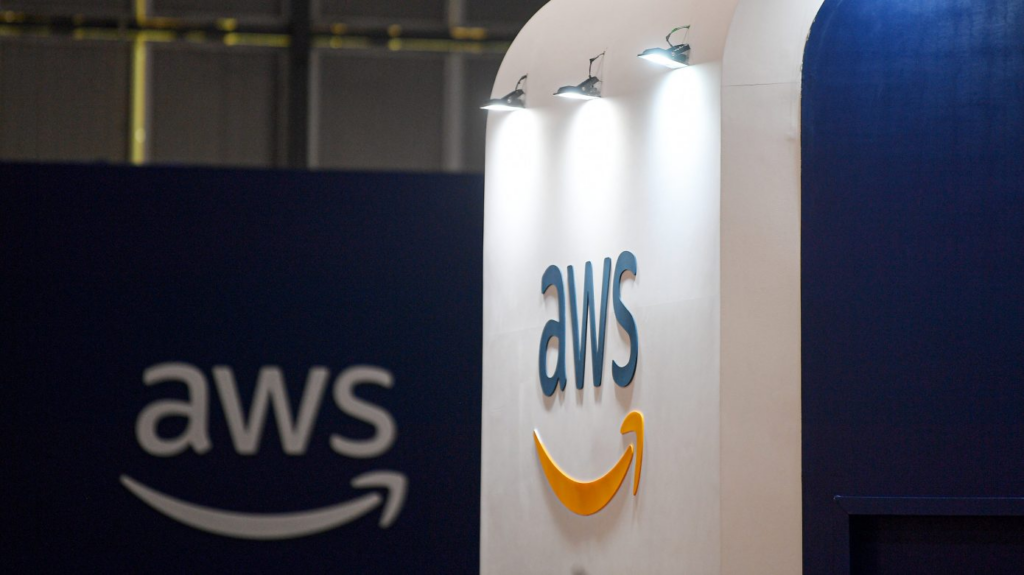
Amazon Unveils New Chips for Training [Source of Image: Techcrunch.com]
AWS clients can only expect Trainium2 instances “sometime next year,” according to Amazon. You may be confident that we’ll be watching for updates.
Amazon unveiled the Arm-based Graviton4, its second chip, for inferencing this morning. It is different from Amazon’s other inferencing chip, Inferentia, and is the fourth generation in the Graviton chip family (as shown by the “4” added to “Graviton”).
According to Amazon, when running on Amazon EC2, Graviton4 offers up to 30% greater compute performance, 50% more cores, and 75% more memory bandwidth than one Graviton processor from the previous generation, Graviton3 (but not the more current Graviton3E).
Another improvement over Graviton3 is that, according to Amazon, all of Graviton4’s physical hardware interfaces are “encrypted,” thus improving data security and AI training workloads for clients with higher encryption needs. (We’ve spoken with Amazon regarding the precise meaning of “encrypted,” and we’ll update this post accordingly.)
In a statement, Brown said, “Graviton4 is the most powerful and energy-efficient chip we have ever built for a broad range of workloads. It marks the fourth generation we’ve delivered in just five years.” “We can provide our customers with the most cutting-edge cloud infrastructure by concentrating our chip designs on actual workloads that matter to them.”
Amazon EC2 R8g instances, now in preview and will go on sale in the upcoming months, will support Graviton4.
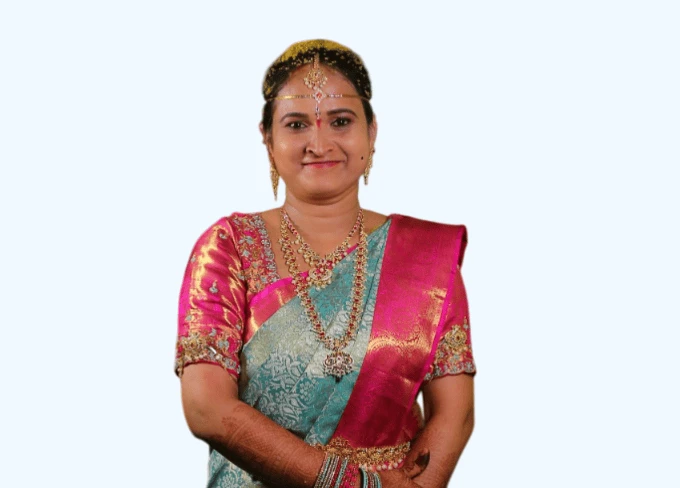
Sai Sandhya is Known for her expertise in Trending News and Startup News. She is passionate about bringing Startup Stories to life. Her proficiency in curating Listings and analyzing Case Studies adds exceptional value to the ever-evolving Business landscape.